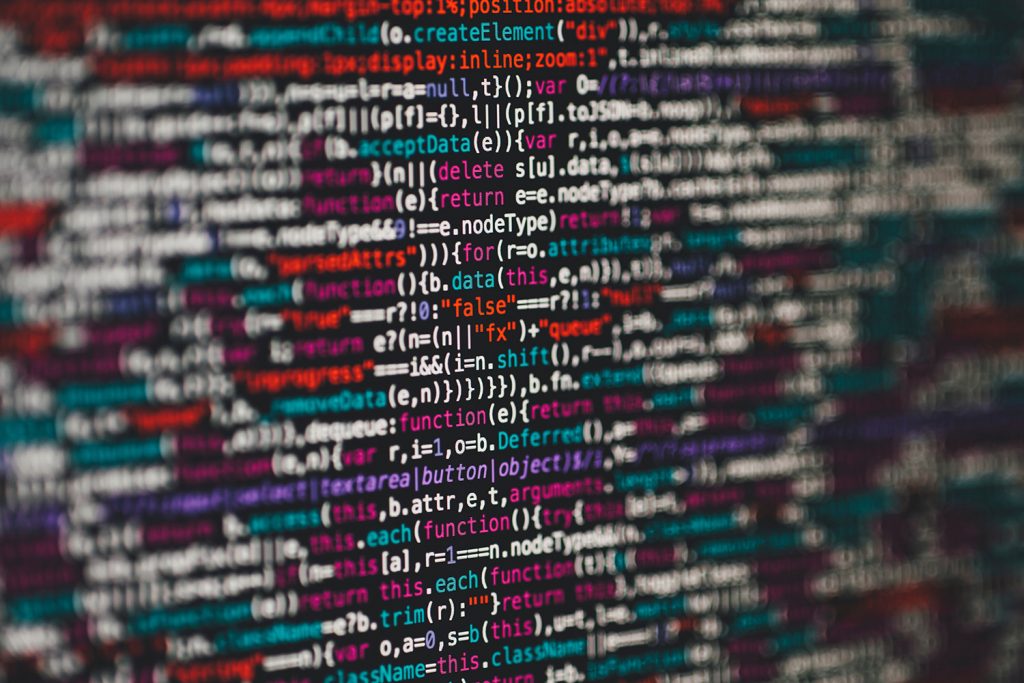
Machine learning and AI are hot priorities for businesses. But there remains a huge gap between AI aspirations and actual execution. In 2020, only 13% of organizations managed to successfully deploy ML use cases in production and plan to keep scaling them. Why such a low number?
In short — because machine learning projects are challenging to productize due to a large number of discrepancies in the ML lifecycle. Granted, that’s changing as Machine Learning as a Service platforms come to the fore.
What is Machine Learning as a Service (MLaaS)?
Whenever there’s a set of repetitive processes, an as-a-service delivery model will inevitably pop up. Understandably, machine learning is no exception.
Machine learning as a service (MLaaS) stands for a standardized setup of cloud-based infrastructure, featuring storage, computing power, supporting tools and libraries, for planning, developing, managing, and deploying ML projects.
Given that data scientists, ML engineers, and everyone else involved constantly need to perform a series of error-prone prep steps for starting a new project, such ML services are designed to streamline menial tasks such as:
- Data cleansing & preparation
- Model training & retraining
- Experiment management
- Model version control
- Run orchestration
- Deployment pipelines
- And more.
The 4 Main Benefits of Machine Learning as a Service Solutions
Per the latest McKinsey data, in 2020 40% of AI leaders used an end-to-end platform for AI-related development projects. So what are they getting out of their investments?
1. Improved Data Management
Good data is key to effective model training and subsequent performance. But data preparation, labeling, and management can eat up a good chunk of productive time. Especially, when the most valuable records are stored deep within on-premise systems.
In that sense, MLaaS platforms offer several benefits:
- Elastic & cost-effective data storage
- Pre-made pipelines for data ingestion
- Toolkits for setting up effective data governance.
Some ML services providers such as Neu.ro also offer data cleansing and labeling services for an extra fee, so that you don’t need to spend time (and cash) on those activities in-house.
2. Ready-to-use ML toolkit
The newer breed of ML platforms come pre-furnished with a staunch range of tools, libraries, notebooks, and frameworks for running machine learning projects. Certain providers also have pre-made APIs for common ML use cases such as predictive analytics, image recognition, and sentiment analysis among others.
Again, having a ready-to-use setup of tools drastically decreases the prep time for every new project and improves scaling abilities. McKinsey estimated that over 48% of AI leaders already use automated tools for developing and testing AI models.
3. Faster Time-to-Productivity
Markets command fast results. But few engineers can catch up. The 2020 Algorithmia survey suggests that over 50% of enterprises need between 8 and 90 days for deploying one model. What’s more 18% of teams spend 90+ days on productionizing a single model. That’s a lot.
Machine learning as a service platforms enable teams to get down to business faster. With suitable infrastructure pre-provisioned and pre-configured, sufficient GPU allocated and necessary pipelines set up, data scientists can focus on what matters most — training, validation, and successful deployments.
4.Lower Total Cost of Ownerships for ML Projects
Computing power is a hot commodity, especially when you constantly need to purchase new and new GPUs to scale your delivery capabilities. Given that a single Nvidia GPU unit costs $699 on average, the TCO bill can rapidly get out of control.
For comparison, cloud TPU can be purchased for as low as $4,50/hour. If you subtract hardware maintenance and electricity costs, the deal gets even better. Also, you’d be only paying for hardware when it’s in use. Lastly, some MLaaS platforms let you run experiments in hybrid environments, mixing on-prem, and cloud resources.
To Conclude
Machine learning as a service may be a new kid in town, but it’s already a popular one. With the benefits outlined above, it makes perfect sense while an increasing cohort of market leaders choose to run their projects on MLaaS platforms, rather than fully in-house.